In our previous article, we discussed the two worlds that we live in simultaneously – the social and the market one; then, we continued our analysis of human action & incentives and how these tie into anchoring, imprinting, and self-herding.
In today’s article, we’ll be looking at how these relate to machine learning, going over a few experiments that tried to understand what makes people buy different things.
In one of his conferences, Rory Sutherland, vice chairman at Ogilvy, said that when faced with a problem, the majority jumps right to the technological path, “nobody is asking for the psychological innovation”. What does that mean?
Whenever a company has a business or customer-related problem, its first instinct is to buy knowledge from a consulting company. It’s behavior that has proved fruitful in the past. So, everyone’s thinking is similar to that of our Starbucks guy, “If it worked in the past, why waste time trying something else?”. It’s a shortcut, and we all use these sometimes. It’s not because we’re lazy, but because we’re psychologically wired not to take risks and get disappointed; we follow the neural path that has already proved its efficiency.
However, there are many instances in business where a problem can be approached from multiple angles. It doesn’t necessarily mean that one is better than the other. Despite being different, they nevertheless provide a totally functional solution.
Understanding market positioning with Rolls Royce
There was a time when Rolls Royce stopped bringing their cars to car shows and started displaying them at aircraft shows. When you place a car in the same area as 98% of all other exhibitors, just seeing it gives you the feeling it’s an expensive hobby.
This is an entirely normal occurrence. When we make decisions, we always take whatever we’re already familiar with or surround us as landmarks. Therefore, they’ve resorted to a trick; they’ve changed the environment and made it fit their products. The effect was immediate.
When surrounded by aircraft priced upwards of 100.000$, paying a tenth of this value on a legacy car would seem like a breeze for your wallet. You’re even tempted to say “I’m not going to buy a jet this year; I’ll take a couple of those Rolls instead”. Bottom line: manipulating the environment can turn the tables in your favor and offer unexpected benefits as it did for Rolls Royce.
Their big idea was not an engineering upgrade but rather psychological innovation. In fairness, until science comes up with a solution to break current technological barriers, like safely driving a car at 500mph, psychological innovation is the one solution we should further explore.
In terms of psychology, we have the disproportionate tendency to value things like white elephant tusks and bears’ heads because we are all biased. We find it challenging to overcome the pattern which states that if something is rare and expensive, it must be automatically valuable.
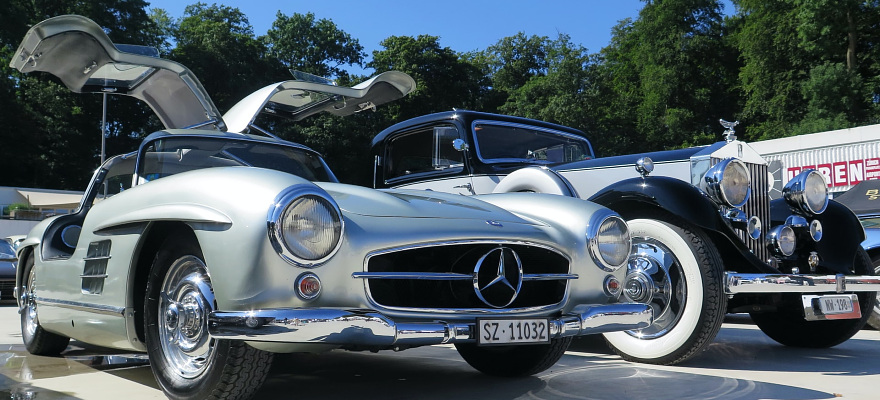
A derived second bias is that inexpensive things are considered less valuable. This mentality has been proven by so many sustainability campaigns that used common commodities in production or involved little to no manual labor.
When faced with such a business competitiveness dilemma, many of us go on autopilot mode: the price for whatever is more expensive should be brought down. However, what happens next is that consumers end up perceiving these alternative products as being less valuable. The autopilot backfires!
A tool for better business decision-making has been in development for quite some time. It’s called machine learning. When combined with behavioral marketing and economics, it can produce outcomes and ideas that nobody would’ve thought possible until now.
To give you an idea of how far machine learning has come, I’m going to share a beyond-funny Target (the mammoth US retailer) story which went viral at the time.
Targeting customers needs
Like any player within the retail market, Target tried to figure out what makes their consumers tick. Having collected a tremendous amount of data, so much that Excel was declared obsolete, they hired a data scientist to compile and analyze said data through a predictive, supervised machine learning process.
One of the prioritized goals was to identify buying patterns in pregnant consumers way before they started shopping for diapers and strollers. The results came out and, based on detailed pregnancy scores, Target thought it would be a good idea to send out coupons to all clients who matched the profiling criteria.
Shortly after the campaign started, a pissed-off man vocally complained that Target is encouraging young people to get pregnant. He went to his nearest Target store, made a scene and everything. When the dust settled a few days after the incident, the man called Target’s customer service and apologized for his reaction. It turns out his daughter was pregnant and due in several months, only he was the last to be informed about it.
Well, as fascinating and promising as this may sound, the process comes with a few social strings attached. Since machine learning offers more and more proof of its extended usability, the data and results are very sensitive to manipulation. If not used ethically, they may end up raising a riot of people imprinted with false assumptions and anchored in negative triggers.
That being said, you can (and should) dip your toes into machine learning practices. When combined with behavioral sciences, the effects will progress exponentially. It can be used to mine large chunks of data and discover variables that influence the apparition of various behaviors. A second usage will be solving problems that require a deeper look into human behavior and fixing some unethical, wrong anti-social assumptions.
To get more practical insights on the potential usage of machine learning in behavioral sciences, scientists from several US universities have used supervised machine learning to identify latent preferences in buying behavior during an experiment on eliciting individual demand.
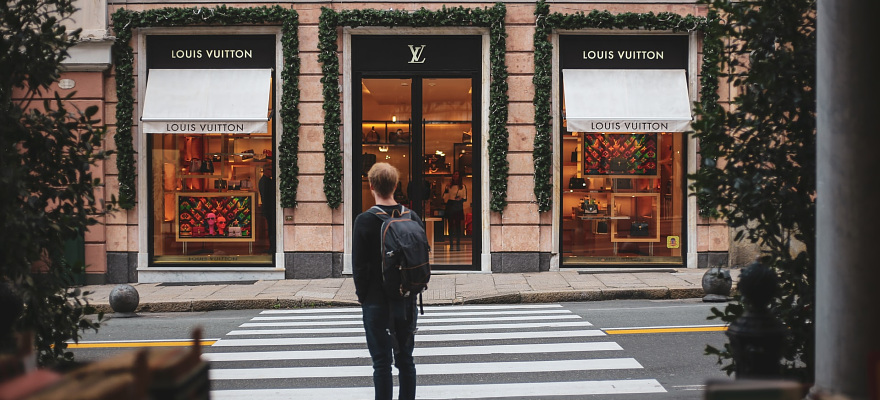
The starting point was to enhance the standard academic direct-elicitation approach for measuring an individual’s valuations for goods procedure (which generates the biased willingness-to-pay values) with supervised machine learning. In other words, they’ve calculated how much were people, in a certain state of mind, valuing specific products and what were they consequently willing to pay.
It may seem counterproductive and some sort of a guessing game, but remember that today’s economic game is no longer anchored solely on pricing strategies, market structures, and technological innovation. It shifted to or, better said, incorporated the psychological side of transactional behaviours.
Economists love looking for all the possible sides of consumers’ latent preferences, such as demand for goods or risk tolerance. Direct elicitation seems to motivate individuals to make choices by revealing their latent preferences.
However, this method maintains validity right now mainly in the theoretical realm rather than the actual marketplace. To improve it and enhance the accuracy of results, researchers used an alternative approach based on supervised machine learning.
The experiment’s subjects were asked to engage in 3 tasks involving choices of food items. The first task was designed to enhance their willingness-to-pay; the second (a binary one) was a two-alternative forced-choice task. The third task was a simple decision on whether to buy a good at a fixed price. The final targeted outcome was developing a standard outcome measure that best represents the kind of decisions that consumers actually make in the marketplace.
Experimenting with economics and choice
Twenty different food items were presented to the subjects. Three food items were common and easy to assess. Each trial took only a few seconds, and all the tasks combined took, on average, less than 30 minutes.
The subjects saw 20 covered cards on the screen and selected two. The cards were flipped over to reveal the bonus items. The subjects received bonus payments of $2 for obtaining a silver item and $4 for getting a gold item. The purpose of these bonuses was two-fold.
Firstly, it checked the subjects’ preferences, as the monetary bonuses should increase willingness-to-pay (it did). Secondly, it generated more significant variation in peoples’ valuations for the food items, greatly increasing the difficulty of making accurate predictions.
The first task elicited their willingness-to-pay for each of the 20 items. It was like getting a price list where each product had multiple prices from which the subjects were to choose one they were willing to pay.
The second task was a two-alternative forced-choice. On each trial, subjects were shown a picture of two items: one placed on the left side of the computer screen and one on the right. Subjects had to decide which of the two items they would prefer to eat, at the end of the experiment, by clicking left or right.
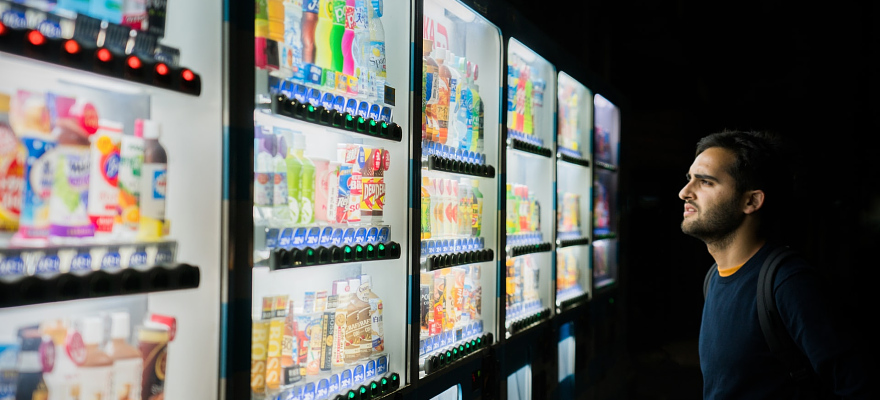
Subjects were asked to choose between all possible pairs of items. The researchers chose this task as they thought it would be one of the simplest, most easily understood ways to get real-choice data. It also represents the most common form of a choice task in all behavioral science.
In the third task, subjects were shown a single food item and its price. They had to decide whether to buy each snack or not, at the posted price. Each snack was offered four times at four different prices.
One of the four prices was the stated willingness-to-pay in the first main task; the other three prices were randomly selected from uniform distributions across low, medium, and high levels. This task is the closest to what consumers experience in real life: a single good listed at a single price with the option to buy or not. The behavior in this task is what they were trying to predict.
The study has concluded that supervised machine learning is an effective alternative for identifying individual-level latent preferences. Compared to the academic approach, it shows that using machine learning helps understand the combination of distinct types of preference-elicitation data, which improves consumer demand predictions. Moreover, the supervised machine learning approach enhances the standard method of predicting purchase decisions.
Final thoughts
Although the combination of machine learning and behavioral economics has wide applications with undeniable benefits, it is still challenged by certain limitations. Some of these limitations include the availability of data for machine learning algorithms and the concerns related to its ethical use.
The success or failure of machine learning applications depends on data availability. If the data is insufficient, to begin with, the machine learning algorithm will not function properly, or the provided results will be limited to the applied sets of data. Secondly, the data used to train the algorithm must be bias-free.
Nudging (the act of encouraging someone to take a specific action) can be unethical. Nudges can be targeted towards a particular set of people or entire populations. However, that can, unfortunately, lead to swarm behavior.
For example, the data can be manipulated so that individuals purchasing items online on an e-commerce platform can be influenced to buy a particular product. This can create bias and lead to financial losses for competitors, as you are intentionally tampering with the market and people’s judgment. It’s best not to deliver nudges at all than to distribute incorrect nudges.
On a much more positive note, the use of machine learning for nudging, when implemented unbiased and within ethical limits, can transform the way individuals make decisions. If proven to be widely effective in future research, machine learning in behavioral economics could help augment human choices in finance, education, investment, and other professional fields.